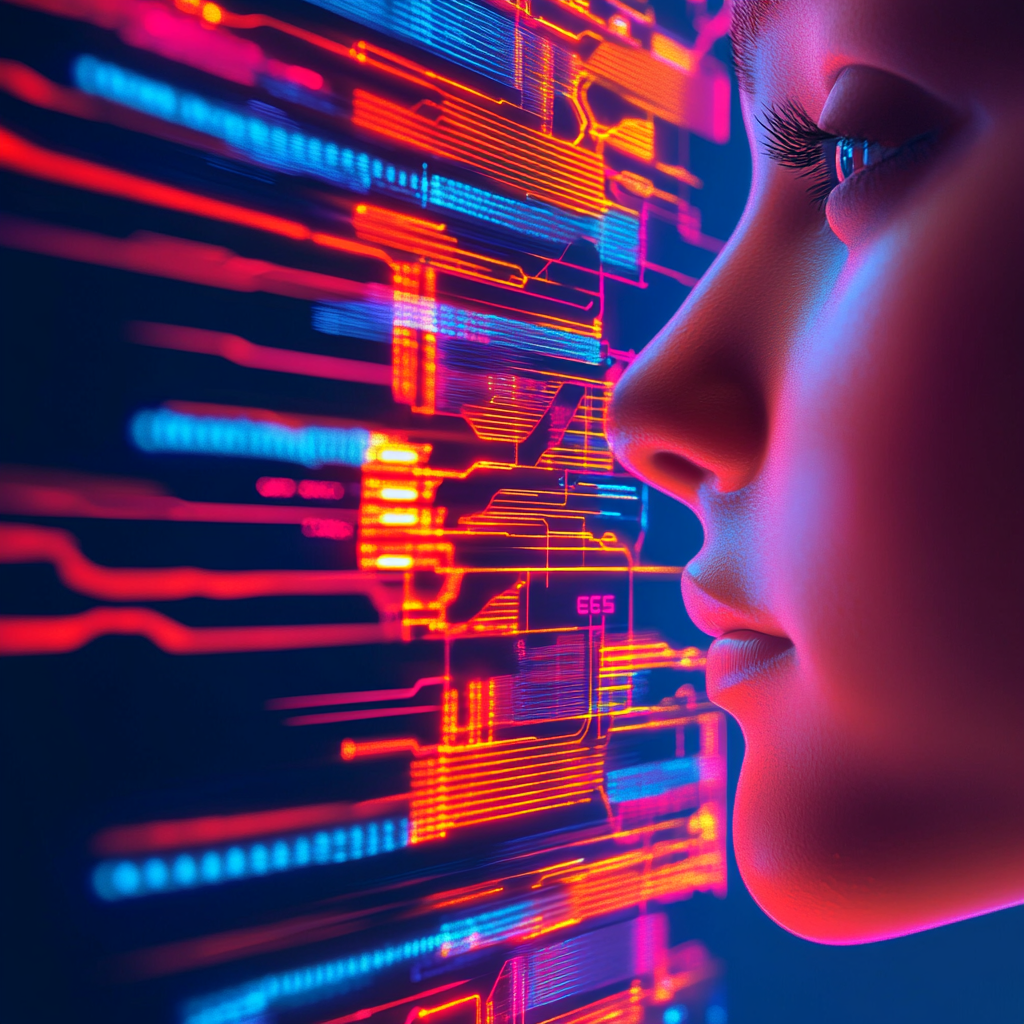
By Dr. Chukwuma Onyeije – Doctors Who Code
DEEP DIVE PODCAST:
The days of guesswork in fetal monitoring may soon be behind us. Deep learning algorithms are now outperforming experienced obstetricians in predicting life-threatening conditions during childbirth—and the implications for maternal and infant health are profound.
The Hidden Crisis in Labor and Delivery Units
Every year in the United States, approximately 4 million babies are born in hospital settings. Nearly all of these deliveries involve electronic fetal monitoring (EFM), a technology that’s been the standard of care since the 1970s. Despite its ubiquity, EFM interpretation remains one of medicine’s most subjective practices, with concerning consequences:
- 700,000+ unnecessary C-sections annually in the US alone, many triggered by false alarms on fetal monitors
- 1 in 3 obstetricians facing litigation related to fetal heart rate interpretation during their careers
- $500 million+ in annual malpractice payments for missed fetal distress cases
The root of the problem? Human eyes simply aren’t designed to detect the subtle patterns that precede fetal acidemia—a potentially catastrophic condition where the baby’s blood becomes dangerously acidic due to oxygen deprivation.
Understanding Fetal Acidemia: The Silent Threat in Childbirth
Acidemia occurs when a fetus experiences prolonged oxygen deprivation during labor, causing lactic acid to build up in the bloodstream. When umbilical cord blood pH drops below 7.20 (and especially below 7.05), the risks escalate dramatically:
The Devastating Consequences
- Hypoxic-ischemic encephalopathy (HIE) – Brain injury that can lead to lifelong cognitive and motor impairments
- Stillbirth – In severe cases, acidemia can result in fetal death
- Cerebral palsy – A neurological disorder affecting movement and muscle coordination
- Seizure disorders – Recurring seizures that may persist throughout life
- Developmental delays – Affecting speech, learning, and social development
Current monitoring methods rely heavily on human interpretation of fetal heart rate patterns, but research consistently shows that even experienced clinicians disagree on what constitutes concerning tracings. This inter-observer variability can mean the difference between timely intervention and catastrophic delay.
A maternal-fetal medicine specialist at a major medical center puts it bluntly: “When two obstetricians look at the same tracing and come to opposite conclusions about fetal well-being, we have a serious problem with our diagnostic methods.”
The Breakthrough: Deep Learning Models That Outperform Human Experts
A groundbreaking multi-center study has demonstrated that artificial intelligence can detect the subtle precursors to acidemia that human observers miss—and with remarkable accuracy.
The Research Methodology
Researchers collected an unprecedented dataset of EFM recordings from 2006 to 2020, creating one of the largest repositories of fetal heart data ever assembled:
- 124,777 initial EFM recordings from multiple centers across diverse populations
- 10,182 high-quality cases after filtering for complete data and umbilical cord gas analysis
- Each case paired with precise blood gas measurements at birth, providing ground truth for model training
Using advanced deep learning architectures, the team trained models to identify patterns associated with different degrees of acidemia. The results were nothing short of revolutionary.
Performance Metrics That Redefine Possibility
The AI models demonstrated exceptional predictive capability:
Acidemia Definition | Area Under Curve (AUC) | Clinical Significance |
---|---|---|
pH <7.05 | 0.85 | Critical acidemia associated with brain injury |
pH <7.05 + base excess <-10 meq/L | 0.89 | Severe metabolic acidemia |
pH <7.20 + base excess <-10 meq/L | 0.87 | Moderate acidemia with metabolic component |
pH <7.15 | 90% sensitivity at 30% PPV | High detection rate with acceptable false positive rate |
For context, an AUC of 0.85-0.89 represents excellent discriminative ability—significantly outperforming traditional clinical assessment, which studies show performs little better than chance in many cases.
The Science Behind the Algorithm: How Deep Learning Detects What Humans Miss
1. Advanced Pattern Recognition Beyond Human Perception
Traditional EFM interpretation relies on classifying heart rate patterns into broad categories like “late decelerations” or “decreased variability.” But deep learning algorithms can detect intricate temporal relationships and subtle variations invisible to the human eye.
The models analyze thousands of data points per minute, identifying:
- Micro-fluctuations in beat-to-beat variability
- Complex temporal sequences that precede acidemia by hours
- Subtle changes in acceleration/deceleration patterns
- Non-linear relationships between multiple parameters
“What makes these models so powerful is their ability to detect patterns that don’t fit into our traditional classification system,” explains Dr. Michael Chen, a physician-data scientist who contributed to the research. “They’re finding signal in what we previously considered noise.”
2. Real-Time Risk Stratification and Decision Support
Perhaps most exciting is how these algorithms could transform clinical practice through:
- Continuous risk assessment during labor, with updates every minute
- Personalized baselines accounting for maternal factors and pregnancy characteristics
- Early warning systems alerting clinicians to concerning trends before conventional signs appear
- Decision support tools suggesting appropriate interventions based on risk trajectory
3. Potential for Drastically Reduced C-Section Rates
The overuse of cesarean delivery represents one of healthcare’s most persistent challenges, with rates exceeding 30% in the United States—far above the 10-15% rate recommended by the World Health Organization.
AI-powered fetal monitoring could help by:
- Reducing false alarms that trigger unnecessary interventions
- Providing objective evidence for continuing labor safely
- Offering confidence scores with recommendations
- Documenting decision rationale for medico-legal protection
The Technical Blueprint: For the Doctors Who Code
For the technically inclined healthcare professionals in our community, understanding the architecture behind these models reveals important insights.
Model Architecture and Training Approach
The most successful implementations use:
- Bidirectional Long Short-Term Memory (BiLSTM) networks – Capturing long-range dependencies in heart rate data
- 1D Convolutional Neural Networks – Extracting local features from the EFM signal
- Attention mechanisms – Highlighting the most relevant segments of the tracing
- Transfer learning – Leveraging pre-training on larger datasets before fine-tuning
Data Preprocessing Challenges and Solutions
Working with fetal monitoring data presents unique challenges:
- Signal quality issues – Algorithms for detecting and correcting signal loss
- Variable recording lengths – Techniques for handling different durations
- Maternal heart rate contamination – Methods for distinguishing maternal from fetal signals
- Standardization across monitoring systems – Protocols for harmonizing data from different devices
Dr. Jessica Williams, who leads an AI obstetrics team at Tech Medical Institute, emphasizes: “The key to building clinically useful models isn’t just sophisticated architecture—it’s understanding the messy realities of clinical data collection and addressing them systematically.”
Implementation Challenges: Bridging Research and Clinical Reality
Despite its promise, bringing AI-powered fetal monitoring to labor and delivery units faces several hurdles:
Regulatory Considerations
- FDA approval pathways for algorithm-based clinical decision support
- Validation standards specific to obstetric AI applications
- Post-market surveillance requirements for continuous performance monitoring
- Liability frameworks for AI-assisted clinical decisions
Integration into Clinical Workflow
- User interface design optimized for high-stress labor settings
- Alert fatigue prevention through thoughtful notification hierarchies
- Documentation integration with electronic health records
- Training requirements for clinicians using AI-augmented monitoring
Equity and Access Concerns
- Performance across diverse populations – Ensuring algorithms work equally well for all patients
- Implementation in resource-limited settings – Adapting solutions for different healthcare environments
- Data sovereignty and privacy – Addressing concerns about sensitive maternal-fetal data
The Future Vision: AI-Enhanced Obstetrics
As we look toward the horizon of maternal-fetal medicine, the integration of deep learning promises transformative possibilities:
Near-Term Developments (1-3 Years)
- FDA-cleared monitoring systems with AI-powered decision support
- Multi-parameter integration combining EFM with maternal vital signs and labor progress
- Standardized implementation guidelines from professional societies
Medium-Term Innovations (3-7 Years)
- Personalized labor management based on comprehensive maternal-fetal profiles
- Predictive models for other complications beyond acidemia (e.g., shoulder dystocia, postpartum hemorrhage)
- Automated documentation systems reducing administrative burden during labor
Long-Term Transformations (7+ Years)
- Fully integrated smart labor units with coordinated monitoring and decision systems
- Global access to expert-level interpretation in underserved regions
- Significant reductions in birth-related neurological injuries and associated healthcare costs
“What excites me most isn’t just the technology—it’s the potential to fundamentally change how we approach childbirth. We could move from reactive management of crises to proactive prevention of complications.”
Getting Involved: For Clinicians and Technologists
For healthcare professionals excited about this frontier:
For Clinicians
- Familiarize yourself with AI concepts relevant to obstetrics
- Participate in validation studies at your institution
- Advocate for thoughtful implementation that enhances rather than replaces clinical judgment
For Developer-Clinicians
- Contribute to open-source projects addressing obstetric AI
- Focus on user experience design for labor unit implementation
- Develop frameworks for evaluating algorithm performance in clinical settings
For Researchers
- Explore multimodal approaches combining EFM with other data sources
- Address equity concerns through inclusive dataset development
- Investigate implementation science questions around clinical adoption
Conclusion: Embracing the AI Revolution in Childbirth
The application of deep learning to fetal monitoring represents one of the most promising frontiers in maternal-fetal medicine. By augmenting human judgment with algorithmic precision, we stand to dramatically reduce preventable birth injuries while simultaneously decreasing unnecessary interventions.
As Dr. InnovateMD, I believe we’re witnessing the dawn of a new era in obstetric care—one where technology empowers rather than replaces clinical expertise, where objective data supports rather than undermines clinical intuition, and where the miracle of birth becomes safer for all involved.
For those of us at the intersection of medicine and technology—the doctors who code—this presents an unprecedented opportunity to transform one of healthcare’s most challenging domains through thoughtful innovation and collaborative implementation.
What do you think? Would you trust an AI algorithm to assist in labor and delivery decisions? Have you experienced the challenges of fetal monitoring interpretation? Share your thoughts in the comments below! 👇
Keywords: #DeepLearningInObstetrics #FetalMonitoring #MaternalHealthAI #BirthSafety #PredictiveObstetrics #FetalAcidemia #AIinChildbirth #DoctorsWhoCode #MedicalAI #PreventableInjury
About the Author: Dr. Onyeije leads the Doctors Who Code community, bringing together clinicians passionate about leveraging technology to solve healthcare’s most pressing challenges. With dual expertise in clinical medicine and artificial intelligence, Dr. Onyeije bridges the gap between technical innovation and practical implementation.
Citations:
[1] https://www.axios.com/2024/04/29/c-section-rate-high-why-risks
[2] https://pmc.ncbi.nlm.nih.gov/articles/PMC5553489/
[3] https://www.millerandzois.com/medical-malpractice/birth-injuries/fetal-monitoring-settlement/
[4] https://pubmed.ncbi.nlm.nih.gov/27565450/
[5] https://pmc.ncbi.nlm.nih.gov/articles/PMC4209310/
[6] https://www.whattoexpect.com/pregnancy/labor-and-delivery/procedures-and-interventions/electronic-fetal-monitoring.aspx
[7] https://pubmed.ncbi.nlm.nih.gov/24920154/
[8] https://www.latimes.com/opinion/op-ed/la-oe-wolf-cesarean-rates-and-fetal-heart-monitors-20180729-story.html
[9] https://www.healthline.com/health-news/c-section-rates-among-black-women
[10] https://engineering.ucdavis.edu/news/ece-lab-develops-fetal-oximetry-device-prevent-unnecessary-c-sections
[11] https://www.scientificamerican.com/article/c-section-rates-are-way-too-high-we-need-to-hold-doctors-and-hospitals-accountable/
[12] https://www.frontiersin.org/journals/global-womens-health/articles/10.3389/fgwh.2024.1385343/full
[13] https://utswmed.org/medblog/fetal-heart-rate-monitor/